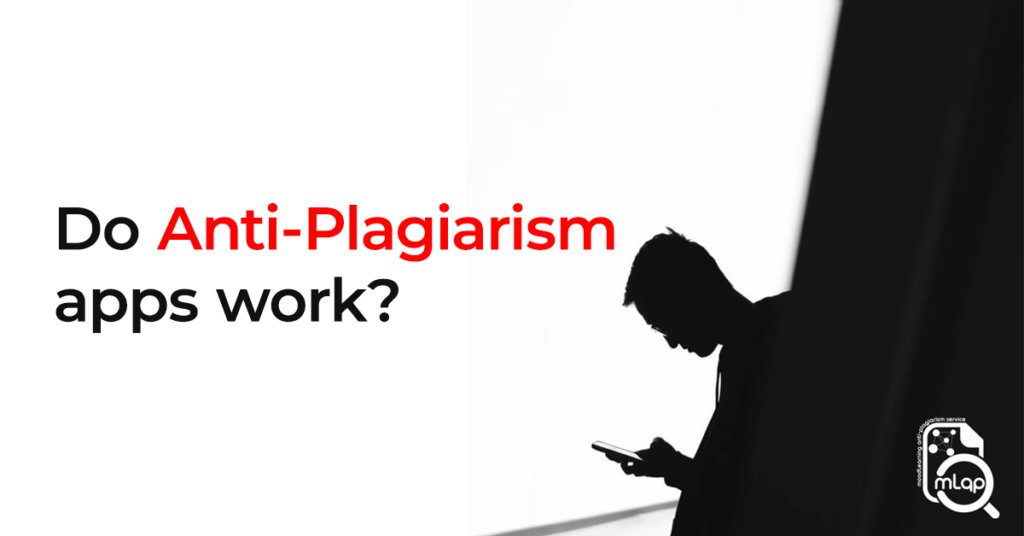
They do, when properly used. Or when users temper their expectations. Consider moodLearning’s anti-plagiarism (mLaP) service, for instance. Like other anti-plagiarism services (including Turnitin), mLaP enables users to upload their articles or assignments, needless to say with no little prodding from instructors. Off they go! One might be forgiven to think that the results of the submission would say, “Oh, plagiarists!” Or, “Oh, congratulations! Not a single plagiarist bone in your body.” The reality, however, is more complicated than that. What the app returns are just similarity results in percentages. Meaning, how your document, or parts of it, resemble with certain documents or parts elsewhere or from the submissions of our your own peers.
So the challenge now is: which percentage would count as definitive in determining plagiarism? Of course there are the obvious ones: 100%, 90%, 80%, and so on. But what about single digits? Or even 11%? The rater, who hopefully knows what he’s doing, would have to go in and take a closer look at the passages or lines concerned.
How much of the similarities could be attributed to malice and deliberate copying? Or to just carelessness?
What could be adding to the challenge of evaluating an article scanned for plagiarism are online paraphrasing tools that makes it easy to cut and paste sentences and paragraphs, transforming them to contents more difficult for plagiarism scanners to recognize as copied materials.
One possible complementary approach is to couple plagiarism scanning with a readability scoring system. The mLaP service does this well, as it juxtaposes scanning with readability scores.
Is the work’s readability level consistent with the author’s or submitter’s readability in previous submissions? One time the submission is “ghetto”; another time, e.e. cummings? One time the essay is grade level 10; another time, graduate school? While this complementary approach is hardly fool-proof, using readability scores to look into “hidden” linguistic structures in submissions helps provide necessary contextual information on scanned materials.
There could be more complementary approaches out there to scan for plagiarism more accurately and holistically. But certainly it cannot just be some mechanical matching of similarity scores, especially for materials with low similarity percentages.
Talk to us at moodLearning if you care about improving plagiarism scanning.
See Also: moodLearning antiPlagiarism Service (mLaP); mLaP Standalone Demo: mlap.moodlearning.com